IndicXNLI: Evaluating Multilingual NLI for Indian Languages
About
While Indic NLP has made rapid advances recently in terms of the availability of corpora and pre-trained models, benchmark datasets on standard NLU tasks are limited. To this end, we introduce IndicXNLI, an NLI dataset for 11 Indic languages. It has been created by high-quality machine translation of the original English XNLI dataset and our analysis attests to the quality of IndicXNLI. By finetuning different pre-trained LMs on this IndicXNLI, we analyze various cross-lingual transfer techniques with respect to the impact of the choice of language models, languages, multi-linguality, mix-language input, etc. These experiments provide us with useful insights into the behaviour of pre-trained models for a diverse set of languages.
tldr: INDICXNLI is an NLI dataset for 11 Indian languages. We investigate the impact of different finetuning strategies, languages, multi-linguality and mixed-language input on various pre-trained language models using the dataset.
People
The following people have worked on the paper, "IndicXNLI: Evaluating Multilingual NLI for Indian Languages":
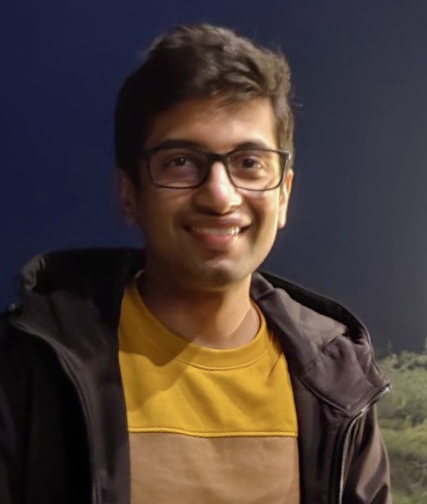
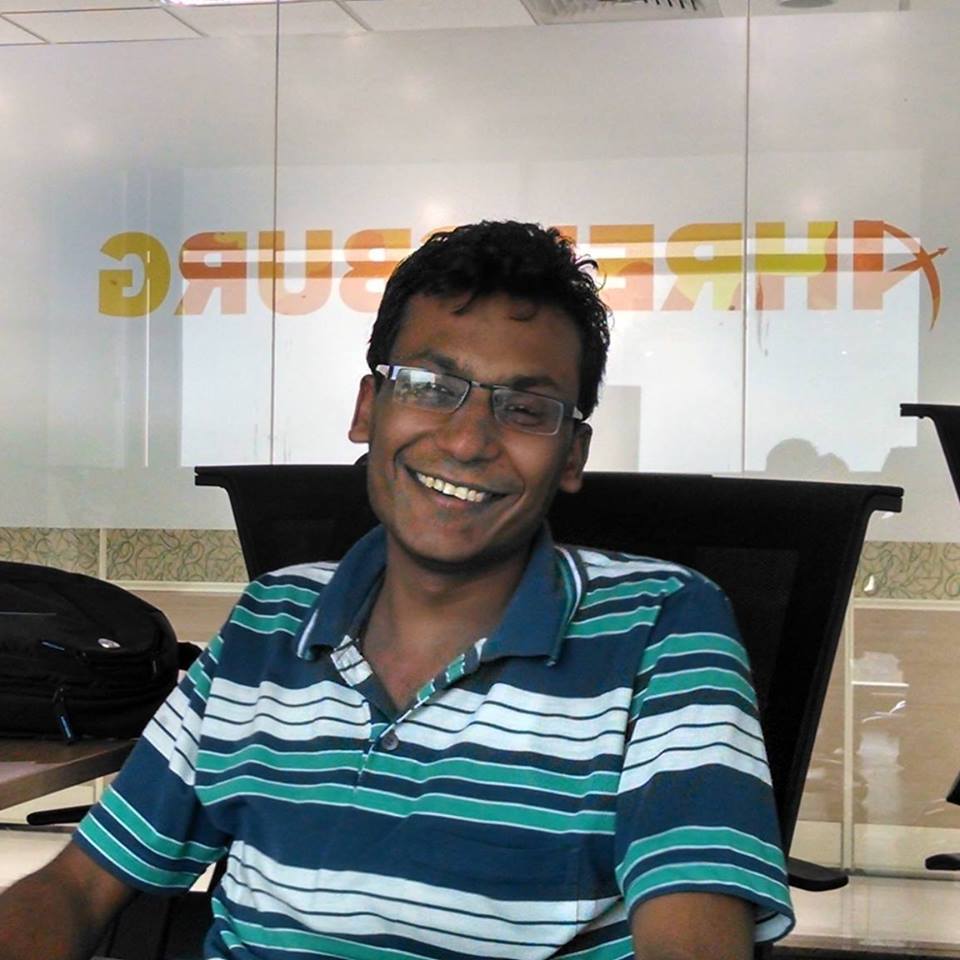
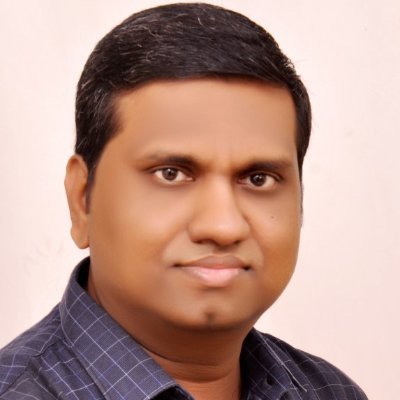
Citation
Please cite our paper as below.
@inproceedings{aggarwal-etal-2022-indicxnli,
title = "{I}ndic{XNLI}: Evaluating Multilingual Inference for {I}ndian Languages",
author = "Aggarwal, Divyanshu and
Gupta, Vivek and
Kunchukuttan, Anoop",
booktitle = "Proceedings of the 2022 Conference on Empirical Methods in Natural Language Processing",
month = dec,
year = "2022",
address = "Abu Dhabi, United Arab Emirates",
publisher = "Association for Computational Linguistics",
url = "https://aclanthology.org/2022.emnlp-main.755",
pages = "10994--11006",
abstract = "While Indic NLP has made rapid advances recently in terms of the availability of corpora and pre-trained models, benchmark datasets on standard NLU tasks are limited. To this end, we introduce INDICXNLI, an NLI dataset for 11 Indic languages. It has been created by high-quality machine translation of the original English XNLI dataset and our analysis attests to the quality of INDICXNLI. By finetuning different pre-trained LMs on this INDICXNLI, we analyze various cross-lingual transfer techniques with respect to the impact of the choice of language models, languages, multi-linguality, mix-language input, etc. These experiments provide us with useful insights into the behaviour of pre-trained models for a diverse set of languages.",
}
Acknowledgement
Authors thank members of the Utah NLP group for their valuable insights and suggestions at various stages of the project, and reviewers for their helpful comments. Additionally, we appreciate the inputs provided by Vivek Srikumar and Ellen Riloff. Vivek Gupta acknowledges support from Bloomberg's Data Science Ph.D. Fellowship.